Catalogue Search | MBRL
نتائج البحث
MBRLSearchResults
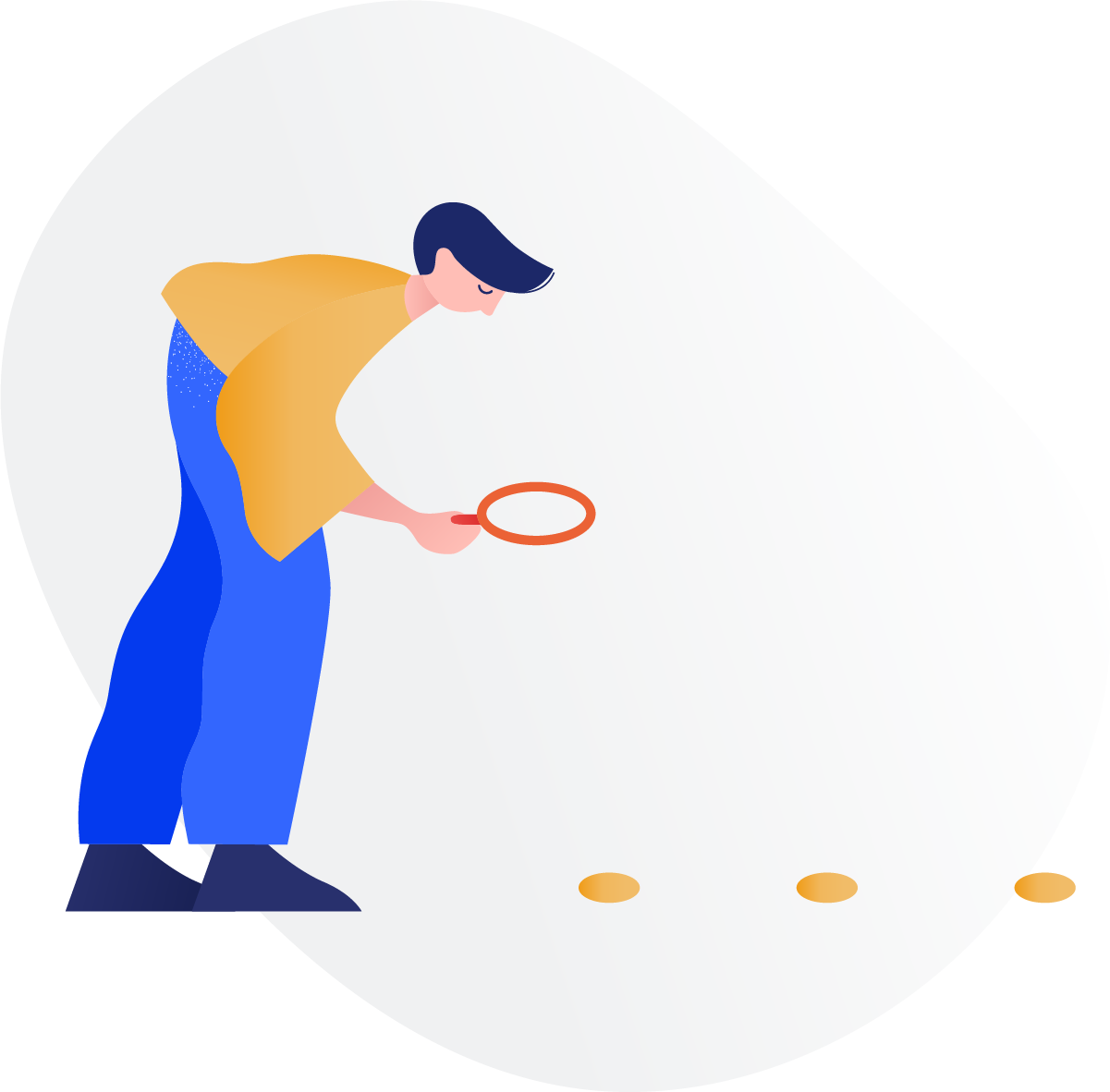
وجه الفتاة! هناك خطأ ما.
أثناء محاولة إضافة العنوان إلى الرف ، حدث خطأ ما :( يرجى إعادة المحاولة لاحقًا!
-
الضبطالضبط
-
مُحَكَّمةمُحَكَّمة
-
نوع العنصرنوع العنصر
-
الموضوعالموضوع
-
السنةمن:-إلى:
-
المزيد من المرشحاتالمزيد من المرشحاتالمصدراللغة
منجز
مرشحات
إعادة تعيين
10,802
نتائج ل
"Autoregressive models"
صنف حسب:
LIKELIHOOD INFERENCE FOR A FRACTIONALLY COINTEGRATED VECTOR AUTOREGRESSIVE MODEL
بواسطة
Johansen, Søren
,
Nielsen, Morten Ørregaard
في
Applications
,
Asymptotic value
,
Autoregressive models
2012
We consider model based inference in a fractionally cointegrated (or cofractional) vector autoregressive model, based on the Gaussian likelihood conditional on initial values. We give conditions on the parameters such that the process X t is fractional of order d and cofractional of order d — b; that is, there exist vectors β for which βʹX t is fractional of order d — b and no other fractionality order is possible. For b = 1, the model nests the I(d — 1) vector autoregressive model. We define the statistical model by 0 < b ≤ d, but conduct inference when the true values satisfy 0 ≤ d₀ — b₀ < 1/2 and b₀ ≠ 1/2, for which ${{\\mathrm{\\beta }}^{\\prime }}_{0}{\\mathrm{X}}_{\\mathrm{t}}$ is (asymptotically) a stationary process. Our main technical contribution is the proof of consistency of the maximum likelihood estimators. To this end, we prove weak convergence of the conditional likelihood as a continuous stochastic process in the parameters when errors are independent and identically distributed with suitable moment conditions and initial values are bounded. Because the limit is deterministic, this implies uniform convergence in probability of the conditional likelihood function. If the true value b₀ > 1/2, we prove that the limit distribution of ${\\mathrm{T}}^{{\\mathrm{b}}_{0}}(\\hat{\\mathrm{\\beta }}-{\\mathrm{\\beta }}_{0})$ is mixed Gaussian, while for the remaining parameters it is Gaussian. The limit distribution of the likelihood ratio test for cointegration rank is a functional of fractional Brownian motion of type II. If b₀ < 1/2, all limit distributions are Gaussian or chi-squared. We derive similar results for the model with d = b, allowing for a constant term.
Journal Article
Short-Term Electricity Prices Forecasting Using Functional Time Series Analysis
بواسطة
Ali, Sajid
,
Jan, Faheem
,
Shah, Ismail
في
Algorithms
,
Artificial intelligence
,
Autoregressive models
2022
In recent years, efficient modeling and forecasting of electricity prices became highly important for all the market participants for developing bidding strategies and making investment decisions. However, as electricity prices exhibit specific features, such as periods of high volatility, seasonal patterns, calendar effects, nonlinearity, etc., their accurate forecasting is challenging. This study proposes a functional forecasting method for the accurate forecasting of electricity prices. A functional autoregressive model of order P is suggested for short-term price forecasting in the electricity markets. The applicability of the model is improved with the help of functional final prediction error (FFPE), through which the model dimensionality and lag structure were selected automatically. An application of the suggested algorithm was evaluated on the Italian electricity market (IPEX). The out-of-sample forecasted results indicate that the proposed method performs relatively better than the nonfunctional forecasting techniques such as autoregressive (AR) and naïve models.
Journal Article
Wind power prediction based on variational mode decomposition multi-frequency combinations
بواسطة
LIU, Hongchi
,
WU, Chen
,
ZHANG, Jiangbin
في
Accuracy
,
Aliasing
,
Artificial neural networks
2019
Because of the uncertainty and randomness of wind speed, wind power has characteristics such as nonlinearity and multiple frequencies. Accurate prediction of wind power is one effective means of improving wind power integration. Because the traditional single model cannot fully characterize the fluctuating characteristics of wind power, scholars have attempted to build other prediction models based on empirical mode decomposition (EMD) or ensemble empirical mode decomposition (EEMD) to tackle this problem. However, the prediction accuracy of these models is affected by modal aliasing and illusive components. Aimed at these defects, this paper proposes a multi-frequency combination prediction model based on variational mode decomposition (VMD). We use a back propagation neural network (BPNN), autoregressive moving average (ARMA) model, and least squares support vector machine (LS-SVM) to predict high, intermediate, and low frequency components, respectively. Based on the predicted values of each component, the BPNN is applied to combine them into a final wind power prediction value. Finally, the prediction performance of the single prediction models (ARMA, BPNN, LS-SVM) and the decomposition prediction models (EMD and EEMD) are used to compare with the proposed VMD model according to the evaluation indices such as average absolute error, mean square error, and root mean square error to validate its feasibility and accuracy. The results show that the prediction accuracy of the proposed VMD model is higher.
Journal Article
On Non-Linear Non-Gaussian Autoregressive Model with Application to Daily Exchange Rate
بواسطة
Adedotun, A. F.
,
Odekina, G. O.
,
Olatayo, T. O.
في
Anderson Darling
,
Autoregressive model
,
Autoregressive models
2022
Abstract
The most often used distribution in statistical modeling follows Gaussian distribution. But many real-life time series data do not follow normal distribution and assumptions; therefore, inference from such a model could be misleading. Thus, a reparameterized non-Gaussian Autoregressive (NGAR) model that has the capabilities of handling non-Gaussian time series was proposed, while Anderson Darling statistics was used to identify the distribution embedded in the time series. In order to determine the performance of the proposed model, the Nigerian monthly exchange rate (Dollar-Naira Selling Rate) was analyzed using proposed and classical autoregressive models. The proposed model was used to determine the joint distribution of the observed series by separating the marginal distribution from the serial dependence. The maximum Likelihood (MLE) estimation method was used to obtain an optimal solution in estimating the generalized gamma distribution of the proposed model. The selection criteria used in this study were Akaike Information Criterion (AIC). The result revealed through the value of the Anderson Darling statistics that the data set were not normally distributed. The best model was selected using the minimum values of AIC value. The study concluded that the proposed model clearly shows that the non-Gaussian Autoregressive model is a very good alternative for analyzing time series data that deviate from the assumptions of normality and, in particular, for the estimation of its parameters.
Journal Article
Prediction and Forecasting of Maximum Weather Temperature Using a Linear Autoregressive Model
بواسطة
Al-Bdairi, Nabeel Saleem Saad
,
Zubaidi, Salah L.
,
Hashim, Khalid
في
Autoregressive Model
,
Autoregressive models
,
Autoregressive processes
2021
Abstract
This paper investigates the autoregressive (AR) model performance in prediction and forecasting the monthly maximum temperature. The temperature recordings are collected over 12 years (i.e., 144 monthly readings). All the data are stationaries, which is converted to be stationary, via obtaining the normal logarithm values. The recordings are then divided into 70% training and 30% testing sample. The training sample is used for determining the structure of the AR model while the testing sample is used for validating the obtained model in forecasting performance. A wide range of model order is selected and the most suitable order is selected in terms of the highest modelling accuracy. The study shows that the monthly maximum temperature can accurately be predicted and forecasted using the AR model.
Journal Article
Highly public anti-Black violence is associated with poor mental health days for Black Americans
بواسطة
Chae, David H
,
Curtis, David S
,
Washburn, Tessa
في
Aggression
,
Autoregressive models
,
Autoregressive moving average
2021
Highly public anti-Black violence in the United States may cause widely experienced distress for Black Americans. This study identifies 49 publicized incidents of racial violence and quantifies national interest based on Google searches; incidents include police killings of Black individuals, decisions not to indict or convict the officer involved, and hate crime murders. Weekly time series of population mental health are produced for 2012 through 2017 using two sources: 1) Google Trends as national search volume for psychological distress terms and 2) the Behavioral Risk Factor Surveillance System (BRFSS) as average poor mental health days in the past 30 d among Black respondents (mean weekly sample size of 696). Autoregressive moving average (ARMA) models accounted for autocorrelation, monthly unemployment, season and year effects, 52-wk lags, news-related searches for suicide (for Google Trends), and depression prevalence and percent female (for BRFSS). National search interest varied more than 100-fold between racial violence incidents. Black BRFSS respondents reported 0.26 more poor mental health days during weeks with two or more racial incidents relative to none, and 0.13 more days with each log
increase in national interest. Estimates were robust to sensitivity tests, including controlling for monthly number of Black homicide victims and weekly search interest in riots. As expected, racial incidents did not predict average poor mental health days among White BRFSS respondents. Results with national psychological distress from Google Trends were mixed but generally unsupportive of hypotheses. Reducing anti-Black violence may benefit Black Americans' mental health nationally.
Journal Article
Variable selection for spatial autoregressive models with a diverging number of parameters
بواسطة
Xie, Tianfa
,
Du, Jiang
,
Cao, Ruiyuan
في
Asymptotic properties
,
Autoregressive models
,
Autoregressive processes
2020
Variable selection has played a fundamental role in regression analysis. Spatial autoregressive model is a useful tool in econometrics and statistics in which context variable selection is necessary but not adequately investigated. In this paper, we consider conducting variable selection in spatial autoregressive models with a diverging number of parameters. Smoothly clipped absolute deviation penalty is considered to obtain the estimators. Moreover the dimension of the covariates are allowed to vary with sample size. In order to attenuate the bias caused by endogeneity, instrumental variable is adopted in the estimation procedure. The proposed method can do parametric estimation and variable selection simultaneously. Under mild conditions, we establish the asymptotic and oracle property of the proposed estimators. Finally, the performance of the proposed estimation procedure is examined via Monte Carlo simulation studies and a data set from a Boston housing price is analyzed as an illustrative example.
Journal Article
Use of Twitter data to improve Zika virus surveillance in the United States during the 2016 epidemic
بواسطة
Masri, Shahir
,
Li, Chen
,
Jia, Jianfeng
في
Aquatic insects
,
Autoregressive models
,
Calibration
2019
Zika virus (ZIKV) is an emerging mosquito-borne arbovirus that can produce serious public health consequences. In 2016, ZIKV caused an epidemic in many countries around the world, including the United States. ZIKV surveillance and vector control is essential to combating future epidemics. However, challenges relating to the timely publication of case reports significantly limit the effectiveness of current surveillance methods. In many countries with poor infrastructure, established systems for case reporting often do not exist. Previous studies investigating the H1N1 pandemic, general influenza and the recent Ebola outbreak have demonstrated that time- and geo-tagged Twitter data, which is immediately available, can be utilized to overcome these limitations.
In this study, we employed a recently developed system called Cloudberry to filter a random sample of Twitter data to investigate the feasibility of using such data for ZIKV epidemic tracking on a national and state (Florida) level. Two auto-regressive models were calibrated using weekly ZIKV case counts and zika tweets in order to estimate weekly ZIKV cases 1 week in advance.
While models tended to over-predict at low case counts and under-predict at extreme high counts, a comparison of predicted versus observed weekly ZIKV case counts following model calibration demonstrated overall reasonable predictive accuracy, with an R
of 0.74 for the Florida model and 0.70 for the U.S.
Time-series analysis of predicted and observed ZIKV cases following internal cross-validation exhibited very similar patterns, demonstrating reasonable model performance. Spatially, the distribution of cumulative ZIKV case counts (local- & travel-related) and zika tweets across all 50 U.S. states showed a high correlation (r = 0.73) after adjusting for population.
This study demonstrates the value of utilizing Twitter data for the purposes of disease surveillance. This is of high value to epidemiologist and public health officials charged with protecting the public during future outbreaks.
Journal Article
Big Data Analytics and Structural Health Monitoring: A Statistical Pattern Recognition-Based Approach
بواسطة
Sarmadi, Hassan
,
Behkamal, Behshid
,
Mariani, Stefano
في
Autoregressive models
,
Autoregressive moving average
,
Autoregressive moving-average models
2020
Recent advances in sensor technologies and data acquisition systems opened up the era of big data in the field of structural health monitoring (SHM). Data-driven methods based on statistical pattern recognition provide outstanding opportunities to implement a long-term SHM strategy, by exploiting measured vibration data. However, their main limitation, due to big data or high-dimensional features, is linked to the complex and time-consuming procedures for feature extraction and/or statistical decision-making. To cope with this issue, in this article we propose a strategy based on autoregressive moving average (ARMA) modeling for feature extraction, and on an innovative hybrid divergence-based method for feature classification. Data relevant to a cable-stayed bridge are accounted for to assess the effectiveness and efficiency of the proposed method. The results show that the offered hybrid divergence-based method, in conjunction with ARMA modeling, succeeds in detecting damage in cases strongly characterized by big data.
Journal Article
Prediction of Arctic Sea Ice Concentration Using a Fully Data Driven Deep Neural Network
بواسطة
Kim, Hyun-choel
,
Chi, Junhwa
في
arctic sea ice
,
Artificial neural networks
,
autoregressive model
2017
The Arctic sea ice is an important indicator of the progress of global warming and climate change. Prediction of Arctic sea ice concentration has been investigated by many disciplines and predictions have been made using a variety of methods. Deep learning (DL) using large training datasets, also known as deep neural network, is a fast-growing area in machine learning that promises improved results when compared to traditional neural network methods. Arctic sea ice data, gathered since 1978 by passive microwave sensors, may be an appropriate input for training DL models. In this study, a large Arctic sea ice dataset was employed to train a deep neural network and this was then used to predict Arctic sea ice concentration, without incorporating any physical data. We compared the results of our methods quantitatively and qualitatively to results obtained using a traditional autoregressive (AR) model, and to a compilation of results from the Sea Ice Prediction Network, collected using a diverse set of approaches. Our DL-based prediction methods outperformed the AR model and yielded results comparable to those obtained with other models.
Journal Article